6 Reasons Why AI Is a Great Manufacturing Ally
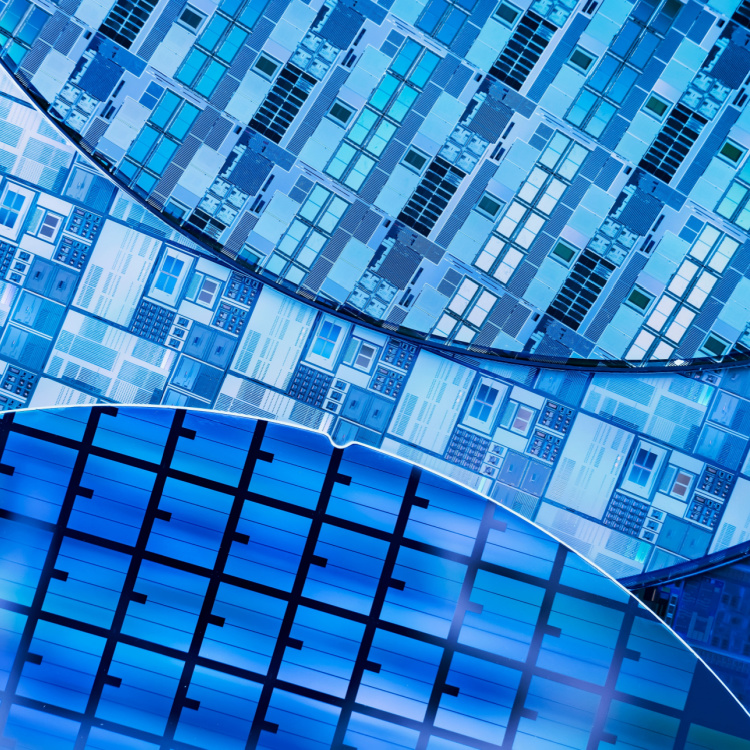
The manufacturing industry constantly searches for new, stable materials for their processes. Fabricating tech essentials, such as chips and batteries, is challenging, especially with supply chain issues. Find out how Google’s AI research laboratory has developed new global manufacturing materials.
Artificial intelligence (AI) holds the capacity to become one of history’s most crucial innovations. Recent proof is Google’s DeepMind, which has discovered hundreds of thousands of new manufacturing materials.
How did this happen? The AI-powered software called GNoME, short for graphical networks for material exploration, used data found on Materials Project, an online database that gives free access to scientific information about materials. As a result, the AI system generated plans for over 2 million new crystals, among which 380,000 are likely to be stable, indicating a lower chance of decomposition or explosive reactions. This means they can be utilized in laboratory experiments, extending the scope of established stable substances by almost ten times. Within this list, it’s possible to identify the next materials for solar cells, superconductors, and solid electrolytes, which can enhance practical technologies.
The journey to discovery
Researchers trained GNoMe via “active learning,” which enabled the software to predict new and stable crystal structures. By going through the entire periodic table, GNoMe produced promising options. Next, a quantum mechanics strategy called density-functional theory (DFT) was used to test and tweak the potential crystals. These revised versions are fed back to the system for deeper refinement.
By repeating the process many times, this technique can produce more complicated structures than the manufacturing industry already uses. Some utilize five or six distinct elements, incorporating multiple atomic interactions that humans didn’t consider.
Still, DFT only validates these structures in the theoretical realm. To see if they can function safely in a real setting, the Lawrence Berkeley National Laboratory created a sub-lab that tests GNoME’s findings and Materials Project data. Experiments are automated, employing machine learning and robots, which can make autonomous decisions on fabricating the material.
Further proof that AI can speed up the exploration and production of materials was how a team randomly selected 58 crystals to fabricate in the laboratory. Initially, the robots failed, which is natural given the trial-and-error nature of experimentation. But by repeatedly tweaking the formulas, the laboratory successfully developed 41 of the materials—a success rate of 71%—after only over two weeks of experiments.
Why AI is ideal for discovering materials
With demand for materials on an uptick, AI can initiate breakthroughs even with constantly changing circumstances. Here are AI’s specific characteristics that enable it to push boundaries and pave the way for revolutionary innovations.
- Working beyond human limitations
Humans need to take breaks, which means 24/7 experimentation and monitoring should be done in shifts, which requires increased staffing. With AI, the software can tirelessly concoct new material recipes and adjust them before real-world testing. Meanwhile, AI-powered robots can work all day and night without posing any safety risk to humans.
- Open-minded approach
Because humans have grown accustomed to specific compositions and ingredients, they may only venture so far from previous discoveries. This “bias” is absent in AI, which can function even with incomplete data and unpredictability. Because AI can pick up patterns within large data sets, it can effectively pinpoint interactions with material compositions and properties, allowing them to form new materials with targeted characteristics.
- Streamlined processes
It takes months to produce promising results from experimenting with new element combinations—and at least a decade to produce something even more viable. With AI coming into the mix, trial periods can be shortened, saving time and money. GNoMe has assembled structures for over 2 million fresh materials, with 700 undergoing lab testing. According to the DeepMind website, the discovery of 2.2 million materials represents about 800 years of knowledge, displaying an impressive scale and precision in predicting.
- Widening possibilities
The widened array of materials boosts opportunities for synthesis and additional data for upcoming AI projects. Discoveries also push scientists and researchers to venture into unexplored areas, possibly giving rise to previously unthinkable possibilities. DeepMind hopes to fast-track innovations in material experimentation by sharing its findings with the research community.
- Simultaneous testing
DeepMind has partnered with outside researchers all over the world. This networking has allowed the independent production of 736 new structures. Laboratory experiments have produced exciting results, such as an optical material with diamond-like properties and a possible superconductor. Because GNoME’s predictions have been opened up to outside researchers, DeepMind hopes to advance inorganic crystal research while unleashing the potential of machine learning tools in experimental processes.
- Sustainability
Materials discovery through AI can expedite breakthroughs in resource management and energy optimization. Algorithms can help identify more energy-efficient synthesis pathways and recyclable materials, promoting a circular economy. AI can also assess the materials’ life cycles, studying their environmental impact from fabrication to discarding. This helps researchers in having a clearer picture of material sustainability.
Challenges of using AI in materials discovery
While the advantages of AI use in this sector are plenty, there are also these downsides:
- Hit-and-miss method
Some argue that using automation to develop new materials isn’t all that practical because researchers typically want to customize their efforts to generate materials with beneficial properties. Letting AI produce thousands without a specific goal may let researchers stray from targets.
- Big numbers, low real results
Though the discovery of 380,000 new materials is impressive, the truth is that only a portion of these are practical to produce. Some require radioactive matter or pricey and scarce elements. Others involve extreme conditions that cannot be safely replicated in a laboratory.
- Lengthy verification process
This isn’t a direct disadvantage of using AI. However, despite the much faster process of materials discovery and fabrication, turning a crystal into an actual product will still take time. Researchers must test crystal components with other materials and modify them with additives multiple times before they are used in product design and development.
Still, the potential role of AI in materials discovery is worth exploring. Despite its downsides, its merits have the capacity to revolutionize the global manufacturing industry–and the devices that have become necessities in our daily lives.
As one of the Top 20 EMS companies in the world, IMI has over 40 years of experience in providing electronics manufacturing and technology solutions.
We are ready to support your business on a global scale.
Our proven technical expertise, worldwide reach, and vast experience in high-growth and emerging markets make us the ideal global manufacturing solutions partner.
Let's work together to build our future today.
Other Blog
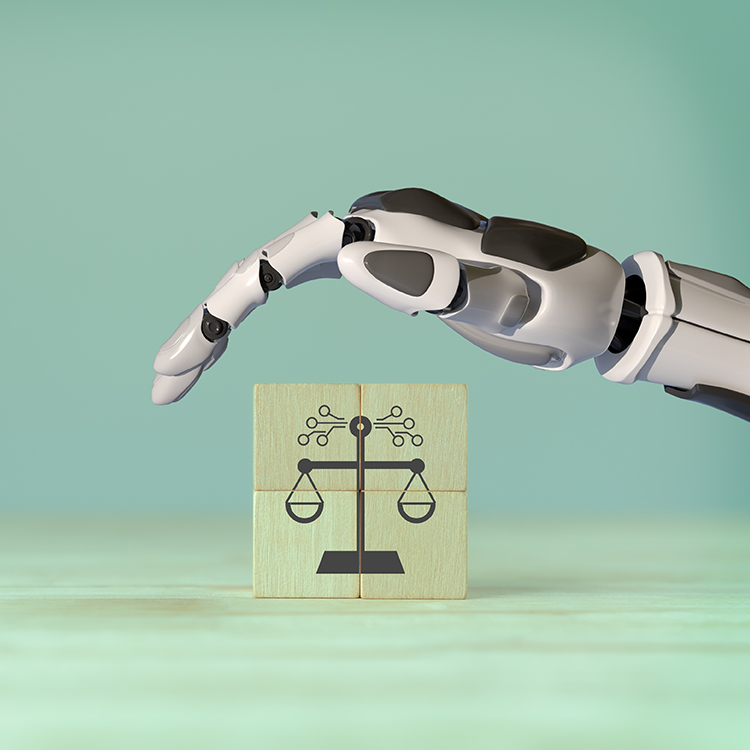
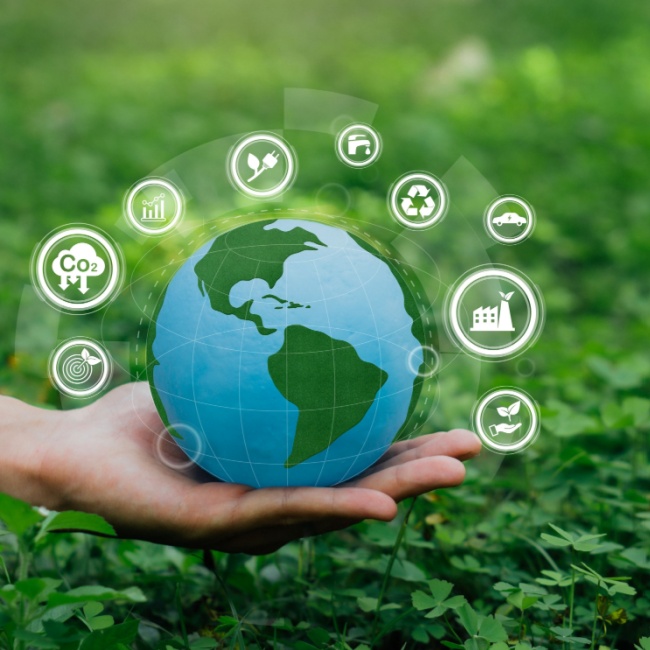
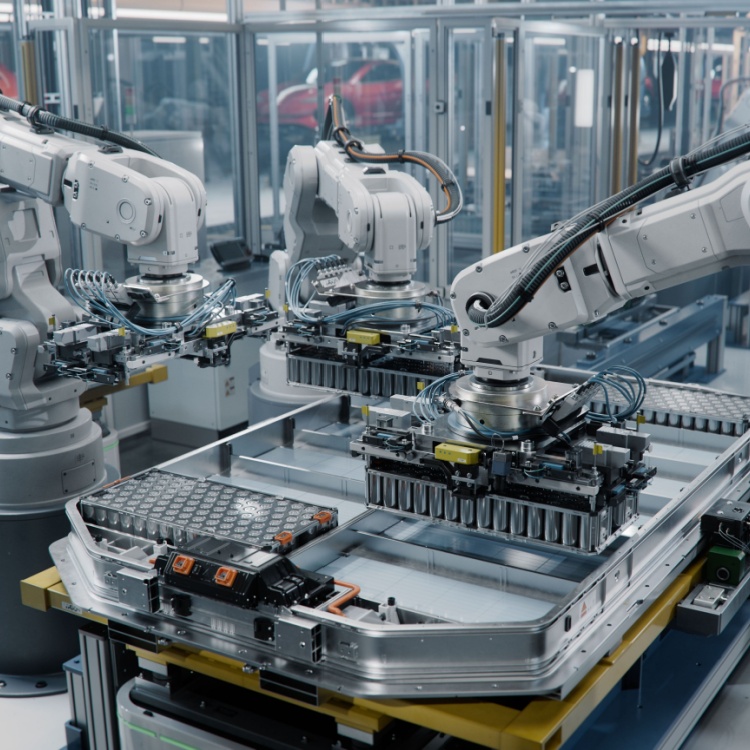